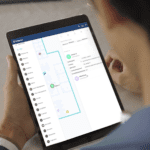
Harnessing the Power of AI for Real Time Location Systems (RTLS)
Enlighted's Artificial Intelligence solutions empower organizations to streamline processes, increase efficiency, and effectively manage assets.
Why Machine learning for RTLS?
In the dynamic world of asset and occupant tracking and management, RTLS have emerged as an indispensable tool for enhancing operational efficiency and optimizing workflows. From helping improve patient experiences in healthcare to tracking forklifts on a busy manufacturing floor, knowing where things are and how they move has value that translates across verticals.
While traditional RTLS provides valuable insights into asset movement, the incorporation of machine learning (ML) takes location prediction to a whole new level of accuracy and intelligence. Not only is it more accurate, but many of the hinderances of traditional RTLS are resolved with an ML-based system. The first, and most relevant to Enlighted’s RTLS Location Intelligence solution, is that the implementation of ML has elevated Bluetooth-based RTLS accuracy to such a level that Bluetooth emerges as a viable, accurate and cost-effective option with endless long-lasting tag variations.
ML can handle the noisy nature of Bluetooth low energy (BLE) signals, the impacts of infrastructure, and strong interference. ML-based RTLS is also more flexible, performing better in the complex and dynamics spaces of our world. Unlike other systems, ML-based RTLS offers the advantage of retraining the ML model when significant changes occur, eliminating the need for gateway or sensor reinstallation and making ML-based RTLS a solution that lasts at a more reasonable price point than other RTLS technologies.
The Foundation of ML-based RTLS
The magic of ML-based RTLS unfolds during the construction of the model. This process lays the foundation of the location engine itself. First, there is the basis of Location Intelligence: the zone definition and zone connection information (how zones are connected). This is when meaningful real-world spaces are translated into zones for the model to generate as predictions. Once defined, data can be collected to capture a fingerprint for each zone.
Data Collection – Quality In, Quality Out
Good data is the cornerstone of any ML model. For the Enlighted solution RTLS BLE data includes received signal strength indicator (RSSI), tag ID, time stamp and sensor ID. This data essentially captures the strength of a certain BLE tag’s beacon signal as perceived by various sensors. Closer sensors will generally detect stronger signals, but that is not always the case due to general noise and interference (e.g. caused by multi-path signals). Moreover, the signal strength may not be uniformly detected by all sensors. The BLE fingerprint encoded in the ML model captures these variations allowing to the advantage of the Enlighted system.
Once collected, this data is labeled with its corresponding zone [Figure A]. This allows the model to learn what RSSI data is recorded by which sensors for a given zone. In fact, when data collection is happening and a tag is being moved throughout a zone, sensors are recording a constant stream of RSSI data as the tag moves, creating a complete picture of the space. To ensure enough data is collected, as quantity is important for an accurate model, many tags are moved throughout the space during this process. For each tag, the data collected by each sensor is patched into one sample. This creates the fingerprint for a tag’s signal in a given zone from the sensors that detected that tag. Once all the data is labeled the model can recognize which patterns are in which zones creating a radio frequency map (BLE fingerprint) of the entire space.
For a scalable data collection and data labeling process, Enlighted has built its own data collection app for streamlining the collection and labeling, and therefore deployment of Location Intelligence. Once a labeled dataset is created, the model can be trained.
Figure A – Example of Data Collection for Model Build in a Zone
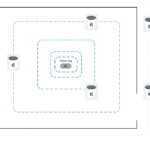
The Model
Enlighted has created its own recurrent neural network (RNN) as the model running in the Location Intelligence engine. The model takes RSSI data collected by the sensors from beaconing tags as an input and outputs a predicted zone. The model is trained to recognize signal patterns and accurately predict from where a tag is beaconing. The model considers the temporal relation between steps since assets move over time (time series data) and an asset’s previously predicted locations are relevant for future predictions.
Training the Model
Once the data is prepared and the layers of the RNN are created, the ground truth data collected on site is used train the model. This involves experimenting with different parameters and evaluating each model based on validation data results. The model is trained over multiple epochs, and the highest performing model is selected. Once a model is selected, it is validated on the test set (dataset that the model has not yet seen) to determine its accuracy when presented with novel data [Figure B].
Once the model is trained and has met accuracy goals, it is deployed.
Figure B – Model Build Process
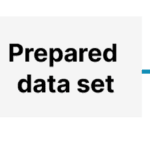
Enlighted Location Intelligence Solution in Action
The Enlighted sensor network receives beacons (BLE signals) emitted from nearby BLE tags when they move or after a specific amount of time spent stationary. The sensors then send signals to the gateways which are connected to the location engine in the cloud [Figure C]. The location engine leverages the deep learning model to predict the location of the tag with 98% zone-level accuracy and a delay of less than 10 seconds.
Figure C – Enlighted Location Intelligence Infrastructure
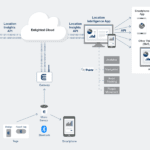
Enlighted serves customer across industries such as healthcare, manufacturing, and commercial offices. Most zones are rooms, defined by their walls and doors. Not only do doors and walls define zones literally, but they also obstruct signals helping create a clear BLE fingerprint. However, in the real world, not all meaningful spaces are defined by walls. Some zones are more complex, such as hallways, curtain divided hospital rooms, and large open manufacturing areas. With our newest version of the Location Intelligence engine, we can predict not only which zone, but where, approximately in the zone a tag is located. The information is passed to an RTLS app, which presents asset and occupant locations, triggers events and alerts for conditions pre-defined by Enlighted customers.
RTLS Accuracy Breeds New Possibilities
The integration of machine learning in RTLS has led to new accuracy and possibilities for RTLS. As these systems continue to learn, adapt, and refine predictions, the use cases and applications continue to grow. From warehouses to healthcare facilities, ML-powered RTLS solutions empower organizations to streamline their processes, increase efficiency, and gain a competitive edge. The future of asset management is here, and it’s powered by the intelligence of AI machine learning.
The brightest way to operate, orchestrate, and optimize everyday spaces.